Why Your Business Needs To Adopt AI
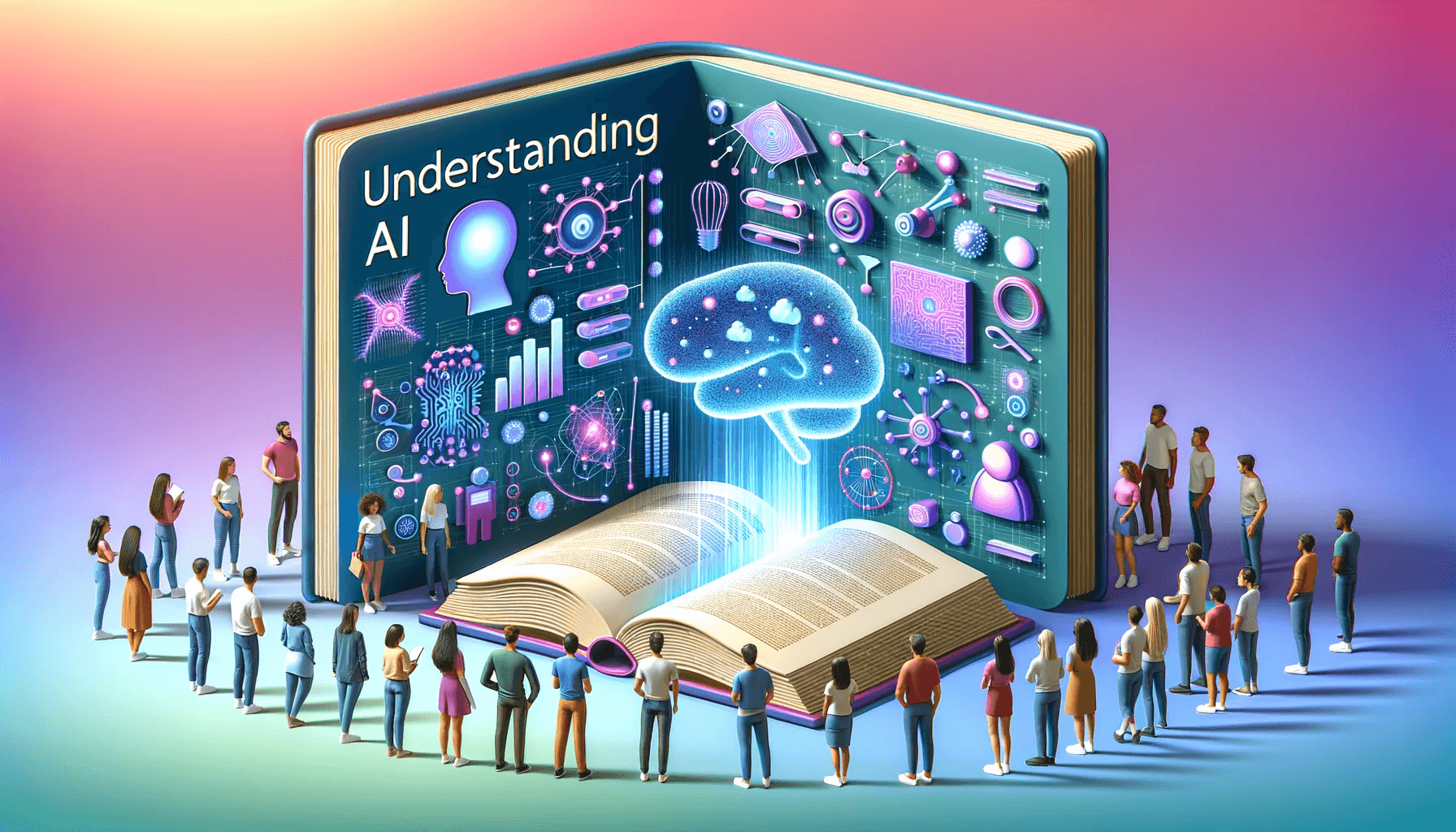
Artificial Intelligence (AI) is completely changing business operations, offering higher efficiency through machine-human interactions. Strategic AI implementation, focusing on real business needs, is the key to beating the competition in today's business environment.
ChatGPT has increased the awareness and utility of artificial intelligence (AI). It has proven that a human can talk to a machine, the machine understands what is said and gives a response that mimics human speech.
Two things happened from ChatGPT's introduction.
Firstly, the large model allowed us to communicate with machines in natural language. This is normal language which is how we speak and write. It’s unstructured. It can interpret language and execute various actions and give you a report of said actions.
Second, it proved that AI can perform intelligently like humans in so many different ways. That means there are multiple ways you can apply it now in business.
For example, AI can do all the redundant tasks that you would hire resources to do. which means you can speed up productivity or improve a process with your laptop and phone.
Not only can AI help with automation, but it can also help with:
- Answering questions from employees about work policies and benefits
- Recommending better, more accurate products
- Creating marketing campaigns
- Detecting fraud
According to IBM, 54% of global organizations are seeing benefits from using AI to automate IT, business or network processes, including cost savings and efficiencies. No matter the type of company you’re running, there are practical applications for AI allover.
As competition increases in the AI space, all businesses must adopt AI at some point.
What Is Artificial Intelligence?
Artificial intelligence (AI) is technology that allows computers to mimic human problem solving capabilities. It is often associated with machine learning and deep learning which are mathematical concepts for creating models.
The most recent advancement in the field is the large language model (LLM), which relies on statistical models to comb through vast amounts of data. Some of the most well-known language models like BERT and LLaMA are open source, enabling developers and small companies to create and leverage AI applications without the high price tag.
“A language model only trained on medical documents might respond very well to inputs related to medical contexts, but be quite bad at responding to other inputs like chitchat or recipes.” ~ Mark Riedl, Computer Scientist
What Are Machine Learning and Deep Learning?
Machine learning is a subset of AI that uses algorithms to analyze patterns and make predictions.
For example, Netflix can recommend new shows for streaming customers based on their viewing history. It might even create music someday.
You could have the AI create music and lyrics for you and mix two genres, like Jay-Z and Rachmaninoff. And the AI will produce new songs instantly, never playing the same song twice.
Deep learning models observe patterns in data and need more data points than machine learning models. For example, you can train deep learning models to classify tree species by their characteristics and identify new species.
What Is a Language Learning Model?
A language learning model is an artificial program that can identify and generate text. It can do this through processing a large amount of data until it can identify patterns in language.
The information used to train a large language module can be as large as millions of gigabytes (petabytes) hence the name "large".
The quality of output by the LLM is dependent on the kind of information used to train the module. One of the most popular LLMs is ChatGPT.
These modules shorten the time needed to complete various tasks or perform them autonomously. Such advancement can lead to eliminating the need for certain manual tasks and reducing the potential for errors.
For example, changing an article’s comprehension level without using an LLM could take four months, whereas LLMs can do the work in minutes.
In another example, Amazon’s Kindle could leverage AI and take every single eBook and turn them into multimedia for the masses. They could create five different books for five different age ranges. They could add illustrations or turn them into a coloring book.
Additionally, with AI, you can take a movie and rerun it with a completely different tone to it. You can rerun it from different characters’ points of view, translate the movie into Spanish or flip the main characters or make five different versions of it.
The possibilities become endless with the application of AI.
What Are Companies Building Right Now?
According to IBM, about 35% of businesses globally are using AI and another 42% are exploring it.
Wix, a website builder, is a notable company building its AI arsenal with style. Wix announced an AI website generator that allows entrepreneurs to build a professional site in minutes. It will even create marketing and social media collateral, if prompted.
Venture capitalists are asking companies to invest in AI. Committees are asking the question, “How can we apply AI?” They know they need it but aren’t sure how to proceed. In the first half of 2023, Pitchfork data revealed that venture capitalists invested $15.2 billion into generative AI companies globally.
Companies use AI to address their specific needs.
For example, a cybersecurity company might leverage AI for anomaly detection or intrusion detection.
Another company might use AI to collect and analyze consumer data to help with decision making.
AI might listen to calls and identify upset people, categorizing and prioritizing them.
There are many ways to use AI and there’s a confusing myriad of available utilities. An agency like Ventive can help identify those needs and resources.

How Can Companies Start Building AI Applications?
Building an app using generative AI requires some understanding of logic and application development.
If you choose to use AI exclusively to develop apps, brace yourself for security holes. You should check compliance with industry standards if you’re in the finance, medical, or engineering industries.
Someone with zero experience working with generative software could use a no-code platform. These platforms make it much easier to interact with the generative AI.
Alternatively, you use AI to narrow down on an idea then get an app development agency to build it.
Using AI isn’t necessary for every business process. Instead, focus on what gives you the highest returns. For example, you could build a feature in your company’s calendar software to allow employees to submit requests for off days instead of using AI.
Risks of Implementing AI (and How to Mitigate Them)
There are few downsides for using AI. Generative AI has a problem with "hallucinations". These models (think ChatGPT, Bard, etc.) are programmed to give you the best answer they can. Unfortunately, the output may not be truthful or accurate.
However, you can train models using internal data to prevent incorrect information from an AI model.
Suppose you’re trying to create a legal document to present in court. You can provide context—have the AI only analyze a set of real cases and respond based on those cases. Then the model will create a far more reliable output that you can use in your research.
“Large Language Models do not have any sense of truth or right or wrong. There are things that we hold to be facts, like the Earth being round. An LLM will tend to say that. But if the context is right, it will also say the opposite because the internet does have text about the Earth being flat. There is no guarantee that a LLM will provide the truth. There may be a tendency to guess words that we agree are true, but that is the closest we might get to making any claims about what an LLM “knows” about truth or right or wrong.” —Mark Riedl
AI also has known security and legal risks. You could create an app that has logical, abstract holes in your product.
For example, you build a product where users can upload pictures, but you failed to add some checks to ensure it doesn't accept explicit images.
Then you release the app.
People start uploading explicit images, the website gets taken down, and lawsuits pour in. Now you’re in court because the AI provided an app that violates state and federal laws.
Additionally, the costs of making an AI application will vary widely depending on what you are trying to do.
You could start by reducing friction points or bottlenecks in your processes, analyze your budget, and understand if you will benefit by using AI. You won’t know until you research it. That way, it will be cheaper and more scalable.
The Bottom Line
AI isn’t a fad. It is disrupting what we create and how we work, accelerating the speed of innovation. We can be far more efficient, helpful, and precise with machines trained to use our internal data. Meet with Ventive and we will help you put AI to use in a model that makes sense for your business.
Glossary
Algorithm: Abundant in digital products and services, algorithms solve specific problems efficiently using mathematics. Think of them as replicating a process like following a recipe or deciding what clothing to wear.
Artificial Intelligence: Computers or robots trained to complete tasks that require human intelligence. Face and voice recognition, chatbots, and credit card fraud detection are some examples.
Deep Learning: A subset of machine learning that helps automate tasks using algorithms. They can process and interpret unstructured data like text, images, or audio, helping humans with classification or transcription tasks. And fraudsters manipulate images, called deepfakes, using deep learning.
Hallucination: A large language model’s tendency to give false or incorrect information in its output due to its programming. An LLM like ChatGPT is trained to respond with confidence, not give 100% accurate responses.
Large Language Model: Trained on billions of parameters, LLMs are deep learning algorithms adept at writing, editing, poetry, image creation, and more.
Machine Learning: Recognizes patterns and mines massive data sets to help solve problems like navigating to a destination, finding a new TV show to watch, and buying a pair of shoes.
Natural Language Processing: To communicate with machines, we must speak the same language. Trained machines understand the way people speak and write and will respond to them. Some examples of NLP include autocomplete, voice assistants, and customer service chatbots.
Want to explore AI for your business? Contact Us to get started.